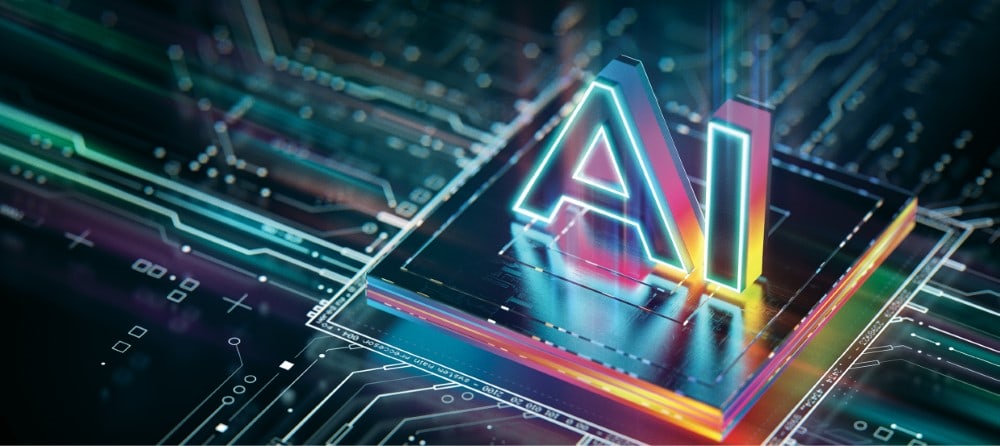
When you speak of artificial intelligence as it relates to aerospace, fields like cyber warfare, cybersecurity, and autonomous weapons systems and drones come to mind. But AI also relates to the more mundane disciplines in the sector. To develop next-generation tech, first you have to win the funding to do so, and that’s gotten significantly more difficult with industry consolidation and a cost-conscious customer. There’s always someone else out there with an excellent technical solution and a stout performance history, so even the most technically robust projects come down largely to cost.
Before it plays a role in developing tech, AI also has a place in business development. Take MEV-II for example, a second-generation “satellite repair-refueling-and-upgrade spacecraft” contemplated by NASA and the USAF.
Using heritage business development practices, our fictional client would select its half-empty San Jose, California, unit for the project based on technical acumen, with a portion of the work likely shifted to its Huntsville, Alabama, subsidiary in a late, desperate attempt to control cost. They would then predictably lose the work to a Houston-based competitor with a full plant (lower overheads), lower labor costs and a lower-cost business climate. Along the way, we and they would convince ourselves we had a winning bid, based on a poorly defined Price-To-Win (PTW), and a self-reassurance that technical promise would win the day (aka “drinking one’s own bathwater” in aerospace speak).
Now let’s try applying a combination of AI and big data to the proposal. The first step is to improve the quality of the PTW. By combining cellphone geography data, census data and various financial databases, we can determine who goes to the competitor’s Houston plant regularly, and back out average labor rates for the facility. Overheads can be derived from FOIA’d heritage proposals or empirically, by analyzing public project and capacity documents. Then procurement can be derived from internal bids adjusted for the make-buy differential, and incorporating the same analysis techniques to major subcontracts as was done with the prime contractor.
Now, before we commit the bid to a heritage facility, we incorporate Analytic Site Selection into the process. We look at the client’s two preferred heritage locations, the competitor’s location (adjusted- our PTW), and a number of greenfield sites. The greenfield sites are determined using additional AI algorithms that scan financial databases throughout the United States looking for localities with detailed labor demographics that match the project requirements, and a significant cost advantage.
AI Works Without Fatigue or Boredom
For MEV-II, the results came back that Pittsburgh, Kansas City, Cincinnati and Indianapolis all offered the required technical skillsets at a reduced cost. However, cross-referencing that data with business climate and incentives records, we determined that a Kansas/Missouri or Ohio/Kentucky partnership would provide a better “incentives-net-business-climate” than Pennsylvania or Indiana. Thus, we kept Cincinnati and Kansas City as the prime greenfield site candidates.
Incentives are often referenced as the “icing on the cake” of a good business deal. In aerospace, they are best considered part of the “cake batter.” Aerospace is a “Core/Base” business engine with significant direct job generation, very high average salaries and a high jobs multiplier. Business incentives are used to enhance the cost savings associated with reduced labor costs by cutting investment requirements and overhead rates, further reducing the bid. And since federal regulation requires such savings be incorporated into the bid, there’s no corporate welfare argument.
So, what do big data parsed and defined by AI tell us? Well, it confirms that a San Jose bid is a likely loss based on the cost premium, and that the Huntsville content merely generates some parity with the competitor’s Houston location. To win on cost by 10%-15% and technical, we might want to look at a new, heavily incentivized location in Cincinnati or Kansas City, bringing some of the San Jose heritage expertise to the bid in the form of relocated or virtual key personnel, or a virtual project office.
But what about fixed overhead coverage at the heritage facilities if we keep breaking out greenfield sites? While we’d argue that workforce constraints are going to force the aerospace sector into a more diversified structure in the future, heritage facilities can still be addressed. It could be that this is just not a good bid for San Jose, and that there is other work better suited to their cost structure. However, it has been my experience that half-empty plants are that way for good reason. It could be that San Jose should change its raison d’etre by disposing of most of its physical plant and consolidating the remaining assets into a smaller, more efficient engineering center of excellence. Such a move would be in line with the principles of total quality management, ensuring that the company’s physical plant structure remains both efficient and effective. Without an analysis defining the plant’s competitive position however, there is no trigger around which to organize change, and you wind up perpetuating inefficiency, resulting in a slow bleed to the plant’s extinction.
The beauty of the AI process is that it isn’t just limited to aerospace. At its core, AI is simply a means of turning a large amount of data reduction over to a series of microprocessors that use self-improving algorithms to sift through mountains of information at incredible speed, without fatigue or boredom, making intelligent decisions and drawing relationships that we are otherwise missing.
The process has equal promise in fields ranging from aerospace to distribution and retail. Want to find a 10% labor advantage in forklift drivers? AI can find them for you. Want to define the demographic information that determines your most successful retail locations and find similar retail locations for expansion? AI can do that for you. Want to find all the locations in the country with the optimum demographics for a data center? AI can do that for you too.
Good fortune and good hunting to you in all your business endeavors.
Geoffrey (Jeff) Troan is a Managing Director at Vista Site Selection LLC, an ancillary company of Vorys, Sater, Seymour and Pease LLP, one of the nation’s largest and oldest law firms. He brings nearly 40 years of business experience to the table, spending more than three decades working in finance, business development and corporate real estate for Lockheed Martin before devoting the last eight years of his career to business and incentives consulting. Contact him at GJTroan@VistaSiteSelection.com.